AI in the Cloud: The Rising Tide of Security and Privacy Risks
Over half of firms adopted AI in 2024, but cloud tools like Azure OpenAI raise growing concerns over data security and privacy risks. As enterprises embrace artificial intelligence (AI) to streamline operations and accelerate decision-making, a growing number are turning to cloud-based platforms like Azure OpenAI, AWS Bedrock, and Google Bard. In 2024 alone, over […]
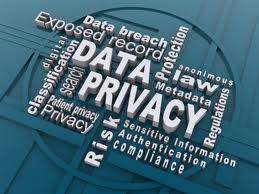
Over half of firms adopted AI in 2024, but cloud tools like Azure OpenAI raise growing concerns over data security and privacy risks.
As enterprises embrace artificial intelligence (AI) to streamline operations and accelerate decision-making, a growing number are turning to cloud-based platforms like Azure OpenAI, AWS Bedrock, and Google Bard. In 2024 alone, over half of organizations adopted AI to build custom applications. While these tools deliver clear productivity gains, they also expose businesses to complex new risks, particularly around data security and privacy.
The Dual Edge of Generative AI
At the heart of modern enterprise AI are generative platforms that power copilots and agents capable of summarizing documents, answering questions, and generating content. Many of these services use techniques like Retrieval-Augmented Generation (RAG), where an AI model dynamically pulls information from knowledge bases or vector databases to provide relevant responses.
But RAG also introduces risk: if access controls are too broad, users may inadvertently (or maliciously) retrieve confidential corporate data. Misconfigured AI agents, for example, might expose sensitive sales reports or customer records to employees who shouldn’t have access.
Misconfigurations and Overexposure
These risks often stem from overly permissive configurations. When AI agents are integrated with enterprise systems, like S3, SharePoint, or Google Drive – it’s essential that their access be governed with strict role-based policies. In one potential scenario, a developer might use an AI copilot intended for Sales and unintentionally access PII or financial data due to lax restrictions.
Custom AI Models Bring Their Own Set of Challenges
Beyond third-party services, many companies build in-house AI and ML models for tasks like credit scoring, fraud detection, or customer personalization. While these models can offer a competitive edge, they pose substantial risks when:
- Sensitive training data isn’t masked or minimized
- Model storage environments aren’t properly secured
- Access controls are poorly defined or unenforced
- Deployed models are exposed to unauthorized users
- “Shadow AI” models go unmonitored, creating blind spots
For instance, a model trained on personal identifiers could inadvertently leak information if not properly governed during training or deployment.
Why Traditional Safeguards Fall Short
Many companies rely on employee training and data handling policies to address these risks. While valuable, these efforts are not enough. Human error is inevitable, and without real-time monitoring and automated controls, sensitive data can still slip through the cracks.
Moving Forward: Principles for Secure AI Use
As AI continues to transform how organizations operate, its adoption must be paired with a proactive and principled approach to data security. This means going beyond basic controls – enforcing granular access, minimizing sensitive data exposure in training pipelines, and continuously monitoring usage to detect misuse or drift. By embracing strong AI data governance practices today, organizations can unlock AI’s full potential while ensuring privacy, compliance, and trust remain at the core of innovation.
About the author: Veronica Marinov, Security Researcher at Sentra.
Follow me on Twitter: @securityaffairs and Facebook and Mastodon
(SecurityAffairs – hacking, privacy)