The Role of Differential Privacy in Protecting Sensitive Information in the Era of Artificial Intelligence
Differential privacy (DP) protects data by adding noise to queries, preventing re-identification while maintaining utility, addressing Artificial Intelligence -era privacy challenges. In the era of Artificial Intelligence, confidentiality and security are becoming significant challenges. Traditional anonymization techniques, such as pseudonymization and k-anonymity, have proven inadequate against sophisticated re-identification attacks. A robust privacy-preserving mechanism called differential […]
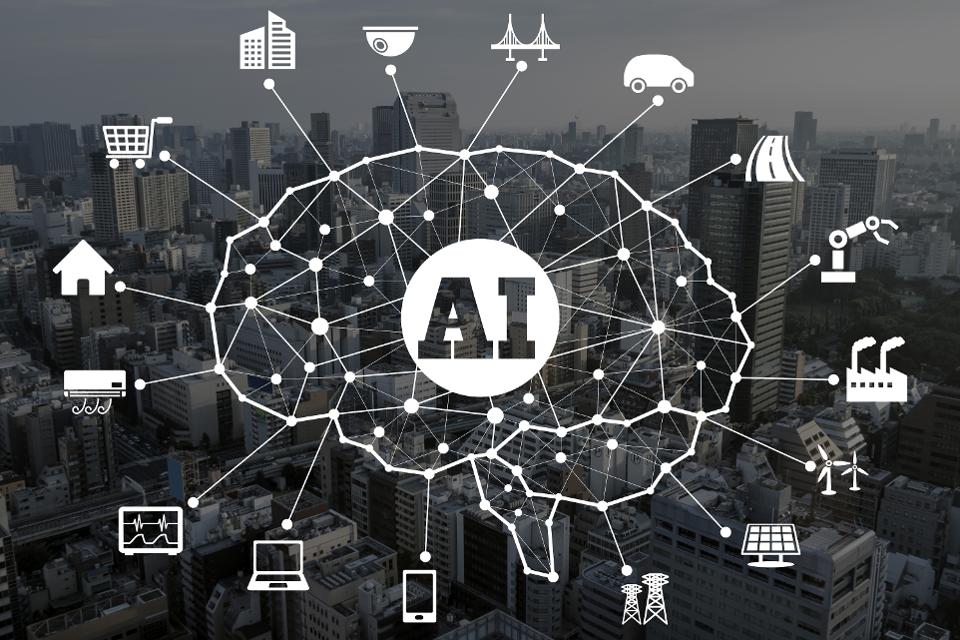
Differential privacy (DP) protects data by adding noise to queries, preventing re-identification while maintaining utility, addressing Artificial Intelligence -era privacy challenges.
In the era of Artificial Intelligence, confidentiality and security are becoming significant challenges. Traditional anonymization techniques, such as pseudonymization and k-anonymity, have proven inadequate against sophisticated re-identification attacks. A robust privacy-preserving mechanism called differential privacy (DP) introduces mathematically guaranteed noise to dataset queries while maintaining statistical utility. This article uses differential privacy in healthcare, finance, and government data analytics to explore the mathematical foundation, implementation strategies, and real-world applications of differential privacy. A comparative analysis with other privacy techniques demonstrates differential privacy’s superior protection. Rising privacy concerns with Artificial Intelligence have paved the way for secure, ethical, and efficient data analysis. Using AI, organizations increasingly rely on data analytics to extract context from vast amounts of information. Concerns over privacy breaches require robust mechanisms to safeguard sensitive user data. Traditional methods of anonymizing data, such as masking and pseudonymization, have been proven inadequate in preventing re-identification attacks. Data privacy has been enhanced by differential privacy (DP), which preserves analytical utility while protecting data privacy. Differential privacy ensures that statistical queries performed on a dataset do not compromise individual privacy, even when an adversary possesses auxiliary knowledge.
A cornerstone in privacy-preserving data analytics introduced the concept of differential privacy, its mathematical basis, and how adding noise ensures privacy. Cynthia Dwork (2006) introduced the fundamental idea, established its mathematical basis, and illustrated how privacy guarantees can be attained by adding numerical work. Cynthia Dwork (2006) introduced the concept of differential privacy, establishing its mathematical foundation and demonstrating how adding noise can achieve privacy guarantees. Their research remains a cornerstone in the field of privacy-preserving data analytics. Their work continues to be a cornerstone in privacy-preserving data analytics.
More recent research has focused on applying differential privacy in various domains. According to Erlingsson (2014), Google’s RAPPOR system collects user data while maintaining anonymity. Similarly, Abowd(2018) examined its integration with a census data collection framework, ensuring confidentiality. Despite its advantages, challenges like keeping data utility and optimizing privacy budgets persist.
Key Concepts of Differential Privacy
Definition and Mathematical Foundation
Differential privacy is mathematically defined using the (ε, δ)-differential privacy model, where ε (epsilon) controls privacy loss and δ (delta) represents the probability of breaking privacy. A mechanism M satisfies ε-differential privacy if: